Yaniel Carreno
Since surface and underwater Autonomous Maritime Systems technology is now mature, the research focus must change to multi-platform coordination and cooperation. This is to achieve persistence, robustness to individual platform failures and improved mission execution, both in terms of timelines but also in terms of the quality of the intelligence gathered. It is also clear that marine drones will shortly be applied to many more applications than the current confines of mine counter measures in the military, data gathering and inspection in the ocean science and off-shore domains. Border security, search and rescue, light intervention and long term monitoring of the ocean will all become areas of growth. In this programme, we propose to explore long-term persistent autonomy for multi-vehicles in the maritime domain. The main aim of the project is to develop algorithms that can devise, execute and monitor plans suitable for long-term missions of marine ‘systems of systems.’ The system must have the ability to adapt its planning and execution of the actions required to carry it out depending on changes in user priority, external conditions, and vehicle availability. Timeline-based planning is especially meaningful in our context as they lend themselves to bridging the gap between task planning and execution monitoring, usually by relying on constraint-based reasoning algorithms. Also, existing implementations of such planners are highly modular and extendible, and have been successfully employed for controlling complex robot systems (e.g. in space missions) In addition, the last generation of these planners can combine several reasoners to account for specifications describing causal, temporal, resource and information dependencies in networked robot systems. However, the inability of modelling unforeseen situations and their reliance on centralised solvers impact their ability to support long-term missions of multiple vehicles in different and changing environments. We will address these problems by investigating the use of multi-agent, decentralised implementations of hierarchical planning using different timelines (weeks, days, hours, minutes). This will be in combination with machine learning approaches to enable long term plans to be adapted effectively. We will also explore how ‘downtime’ in missions can be used opportunistically to carry out other ‘desirable’ actions that are not critical to the mission success. At the lowest level, atomic behaviors will be developed to enable the validation of the system on a number of defence relevant scenarios. These will be combined with safety and survivability ones to develop long term plans. The bandwidth limitation of the communication link between each platform composing the system will limit the amount of data that can be transferred between platforms and the tempo of interactions between the various systems. This will also be taken into account in the planning process. The system will be validated in simulation first to enable the comparison of various techniques and then on real vehicles.
For more information please visit my personal web-page.
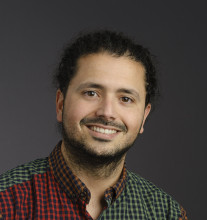