Dr Ioannis Chalkiadakis
Given the success of neural networks in recent years, and especially after the success of deep architectures, their use has been expanding to ever more critical application areas such as security, autonomous driving, and healthcare. Contrary to previous well-understood and thoroughly tested approaches, we still have little understanding of what such machine learning (ML) models learn and when they could fail. The question that naturally arises is whether we can trust such systems to undertake safety-critical tasks. Even though research into rigorous mathematical explanation of popular types of ML models (deep neural networks) is advancing fast, in the light of recent European Union legislation (GDPR, 5/2018) that essentially require accountable models, companies employing such technologies should be able to explain them in an understandable way to non-expert customers, without prior knowledge or expertise in the area.
How can we provide such understanding/interpretation that will allow users to trust the ML model they (indirectly) use, and the model's designers to improve it? This is a crucial question that needs to be considered before we expect people to trust, and hence actually use robotic systems, and is the fundamental research question that we aim to tackle.
Roadmap
The 1st-year MSc. project focused on a sentiment classification task and provided a framework for a data-driven look into the operation of a Long-Short-Term-Memory Recurrent Neural Network. Given the difficulty in defining and measuring the interpretability of neural network models, the evaluation of the latter should initially focus around users, and later on a rigorous evaluation metric. Therefore, we provided a critical evaluation of the framework based on our experience and a pilot study, and concluded by setting the guidelines for a complete user-based evaluation at a future stage.
Starting from this project, our future research continues to be on interpretability in machine learning (ML) models in robotics applications.
Current status and research direction.
Kim (2017 ICML Workshop on Human Interpretability in Machine Learning) asserts that there is no universal method for ML interpretability and our goal should not be to understand every detail but rather provide enough information for the subsequent tasks. She poses three main questions: 1. Why and when is interpretability necessary? 2. What methods can we use to provide explanations? 3. How can we measure the quality of explanations?
Motivated by those questions we identify two research paths:
1) Exploiting the idea of model compression proposed by Ba, Caruana (2014) and further investigated by Hinton et. al. (2015) and Frosst et. al. (2017), we focus on a simple robot navigation task (Freire et. al. 2009) -therefore on a task-specific ML explanation- and aim to instil user trust in the behaviour of the robot (why, what is explanation used for). We believe that expressing the uncertainty in the model's outcome in a user-friendly way will be crucial towards achieving trust, and aim to capture that uncertainty through the compression of the original neural network model used for navigating, to a suitable surrogate model (how to give explanations). 2) Existing approaches for interpreting ML models produce important and helpful results for an expert user, yet what about the non-expert end user? Interpretability will necessarily be achieved with the user target group in mind, however, could we present the expert-oriented interpretations in a way that will be more helpful for non-experts? Regarding uncertainty information, how can experts capture it in machine learning applications, and effectively communicate it to end-users?
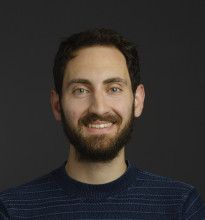