Samuel Garcin
A key limitation preventing the wider adoption of Deep Reinforcement Learning (DRL) today is its difficulty generalising to environments or tasks which were not encountered during training. The frameworks put forward to tackle this issue, such as Meta Reinforcement Learning or Representation Learning, primarily focus on the DRL agent and do not act on the training task generation process. This project will investigate how Representation Learning methods may be employed to learn a structured latent representation of the problem class to be solved, enabling the generation of an adaptive task distribution that captures the problem class, and is adapted to the current level of ability of the agent. The task generator would therefore provide the agent with an adaptive curriculum of tasks, training the agent to generalise to the target problem class.
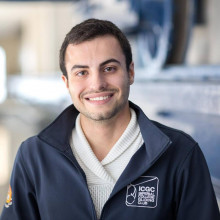