Paulius Dilkas
Given a formula in propositional or (finite-domain) first-order logic and some non-negative weights, weighted model counting (WMC) is a function problem that asks to compute the sum of the weights of the models of the formula. Originally used as a flexible way of performing probabilistic inference on graphical models, WMC has found many applications across artificial intelligence (AI), machine learning, and other domains. Areas of AI that rely on WMC include explainable AI, neural-symbolic AI, probabilistic programming, and statistical relational AI. WMC also has applications in bioinformatics, data mining, natural language processing, prognostics, and robotics. In this work, we are interested in revisiting the foundations of WMC and considering generalisations of some of the key definitions in the interest of conceptual clarity and practical efficiency. We begin by developing a measure-theoretic perspective on WMC, which suggests a new and more general way of defining the weights of an instance. This new representation can be as succinct as standard WMC but can also expand as needed to represent less-structured probability distributions. We demonstrate the performance benefits of the new format by developing a novel WMC encoding for Bayesian networks. We then show how existing WMC encodings for Bayesian networks can be transformed into this more general format and what conditions ensure that the transformation is correct (i.e., preserves the answer). Combining the strengths of the more flexible representation with the tricks used in existing encodings yields further efficiency improvements in Bayesian network probabilistic inference. Next, we turn our attention to the first-order setting. Here, we argue that the capabilities of practical model counting algorithms are severely limited by their inability to perform arbitrary recursive computations. To enable arbitrary recursion, we relax the restrictions that typically accompany domain recursion and generalise circuits (used to express a solution to a model counting problem) to graphs that are allowed to have cycles. These improvements enable us to find efficient solutions to counting fundamental structures such as injections and bijections that were previously unsolvable by any available algorithm. The second strand of this work is concerned with synthetic data generation. Testing algorithms across a wide range of problem instances is crucial to ensure the validity of any claim about one algorithm's superiority over another. However, benchmarks are often limited and fail to reveal differences among the algorithms. First, we show how random instances of probabilistic logic programs (that typically use WMC algorithms for inference) can be generated using constraint programming. We also introduce a new constraint to control the independence structure of the underlying probability distribution and provide a combinatorial argument for the correctness of the constraint model. This model allows us to, for the first time, experimentally investigate inference algorithms on more than just a handful of instances. Second, we introduce a random model for WMC instances with a parameter that influences primal treewidth—the parameter most commonly used to characterise the difficulty of an instance. We show that the easy-hard-easy pattern with respect to clause density is different for algorithms based on dynamic programming and algebraic decision diagrams than for all other solvers. We also demonstrate that all WMC algorithms scale exponentially with respect to primal treewidth, although at differing rates.
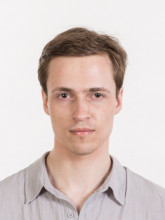