Chris Mower
Construction tasks, such as concrete spraying, demand high levels of concentration and manual dexterity from an operator. Often, these tasks are prolonged and can lead to fatigue and low levels of situational awareness. This can have a damaging impact on safety and has been linked as a contributing factor to the construction sector having one of the highest levels of workplace fatalities - national statistics (Health and Safety Executive, Workplace fatal injuries in Great Britain, 2021) between 2020-2021 reported 39 of the 142 fatalities were from the construction sector (the highest over all sectors). As such, operators require extensive and costly training to perform these tasks at a high quality whilst ensuring minimum safety standards. In the ideal case, regulators would replace this system with an autonomous robot maximizing human safety. However, current AI methods are not able to match, let alone surpass, the contextual awareness and responsive capabilities of skilled human operators.
In order to create a safer environment, improve quality, and reduce costs, this project explores shared autonomy. Shared autonomy aims to combine the natural aptitudes of humans with the latest advancements in multi-modal sensing, the processing capacity of computers, and systematic reasoning abilities of autonomous methods to generate optimal robot motions. Shared autonomy applies not only to construction, but the likes of healthcare, remote inspection, maintenance, disaster recovery, and even space and maritime exploration. The recent developments within this branch of work has generated a number of difficult research questions, that form the focus of this thesis, such as: What kind of assistance would improve task performance without impediment of operator experience? How do we model assistance that would enable a computationally efficient system, responsive enough for online teleoperation in complex environments? And, how can we leverage intention estimation and prediction, and environment sensing, to make predictions of degenerate future events and adapt control strategies early enough to prevent these from becoming a problem?
The work presented in this project, develops methods to address these questions in specific cases inspired by the requirements and restrictions of the construction sector. The direction taken in this work has been to leverage numerical optimization as a framework for modeling shared autonomy that maintains the features of an operators skill and experience, whilst ensuring (changing) physical and operational constraints. We demonstrate the capabilities of the proposed work in realistic lab mock-ups, and evaluate the methods through rigorous, repeatable analysis.
The project is funded by Costain and EPSRC.
Please see my CV. Update: on 29th Sept 2021, I successfully defended my Viva with minor corrections. My examiners were Prof Nick Hawes (University of Oxford) and Dr Michael Herrmann (University of Edinburgh).
Publications
- Christopher E. Mower, Joao Moura, and Sethu Vijayakumar, Skill-based Shared Control, Robotics: Science and Systems (R:SS), 2021.
- Christopher E. Mower, Joao Moura, Aled Davies, and Sethu Vijayakumar. Modulating Human Input for Shared Autonomy in Dynamic Environments. The 28th IEEE International Conference on Robot & Human Interactive Communication (RO-MAN), New Delhi, India (2019).
- Christopher E. Mower, Wolfgang Merkt, Aled Davies, and Sethu Vijayakumar. Comparing Alternate Modes of Teleoperation for Constrained Tasks . Proc. 15th IEEE Conference on Automation Science and Engineering (CASE), Vancouver, Canada (2019). preprint
- Wolfgang Merkt, Yiming Yang, Theodoros Stouraitis, Christopher E. Mower, Maurice Fallon, and Sethu Vijayakumar, Robust Shared Autonomy for Mobile Manipulation with Continuous Scene Monitoring, Proc. 13th IEEE Conference on Automation Science and Engineering (CASE), Xian, China (2017).
Education:
MSc Computing (Visual Information Processing) from Imperial College London.
MSc Applied Mathematics with Numerical Analysis from The University of Manchester.
BSc Mathematics from the University of Sheffield.
Notable Experience:
During my time at The University of Manchester (UoM) I contributed code to the Numerical Algorithms Group (NAG) Library in collaboration with Dr Craig Lucas (NAG), Prof. Nicholas J. Higham (UoM), and Dr Natasa Strabic (UoM). I authored the function named G02ANF in the Fortran programming language, see also here. For an estimated and potentially invalid correlation matrix, G02ANF returns a valid correlation matrix subject to fixing a leading principle submatrix by applying the smallest uniform perturbation to the remainder of the input while preserving the unit diagonal. The method implements a shrinking algorithm developed by Higham, Strabic, and Sego; see paper. My dissertation, entitled Shrinking For Restoring Definiteness, was based on this work and sponsored by NAG.
Whilst at Imperial College London, I worked on two projects with The Hamlyn Centre. The first was part of an Independent Study Option (ISO) with Dr Su-Lin Lee entitled Learning Catheter Paths For Cardiac Mapping that explored assistive methods based on vision and mappings of the heart chambers for catheter ablation procedures. The second was my final dissertation supervised by Dr Benny Lo that explored machine learning methods, such as Hidden Markov Models, to ascertain the skill level for a surgeon using TI Sensor Tags attached to the surgeons wrists during laparoscopic training procedures. Both works were inspired by recent developments in the Internet of Things (IOT) and Body Sensor Networks.
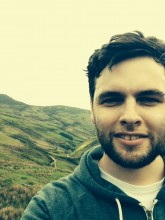